일 | 월 | 화 | 수 | 목 | 금 | 토 |
---|---|---|---|---|---|---|
1 | 2 | 3 | 4 | 5 | ||
6 | 7 | 8 | 9 | 10 | 11 | 12 |
13 | 14 | 15 | 16 | 17 | 18 | 19 |
20 | 21 | 22 | 23 | 24 | 25 | 26 |
27 | 28 | 29 | 30 |
- woodbury matrix identity
- detection
- AERIAL IMAGE
- kakao map
- Map
- pytorch
- dataset
- rotated RetinaNet
- gstreamer
- 영상처리
- bernoulli distribution
- MSAC
- entropy
- measure
- Poisson Distribution
- schur complement
- CoCo
- RANSAC
- matlab
- binomial distribution
- OpenMMlab
- LDU decomposition
- vision
- probability
- MMrotate
- similarity
- DataLorder
- Today
- Total
끊임없이 부단히
MATLAB으로 간단한 RANSAC 구현하기 본문
이번 포스팅에서는 이상치(outlier) 제거에 자주 사용되는 RANSAC 알고리즘을 구현하려고 합니다.
영상처리에서는 특히 뷰 포인트가 다른 두 영상을 매칭할 때 자주 사용됩니다.
(정확히는 특징점을 추출하고 특징점들로 호모그래프를 도출할 때 이상치들을 제거합니다.)
하지만 본 글에서는 MATLAB을 이용하여 간단한 예제만 구현하려고 합니다.
본 코드는 MATLAB 예제 코드를 참고하였습니다.
https://kr.mathworks.com/help/vision/ref/ransac.html
먼저, 임의의 데이터를 생성해줍니다.
기본적으로 y = 2x라는 관계를 같지만, 일부를 난수로 하여 이상치로 만듭니다.
x = 1:0.5:20;
y = 2*x;
errIdx = 1:4:length(x);
y(errIdx) = 20*rand(1,length(errIdx));
plot(x, y, 'o');
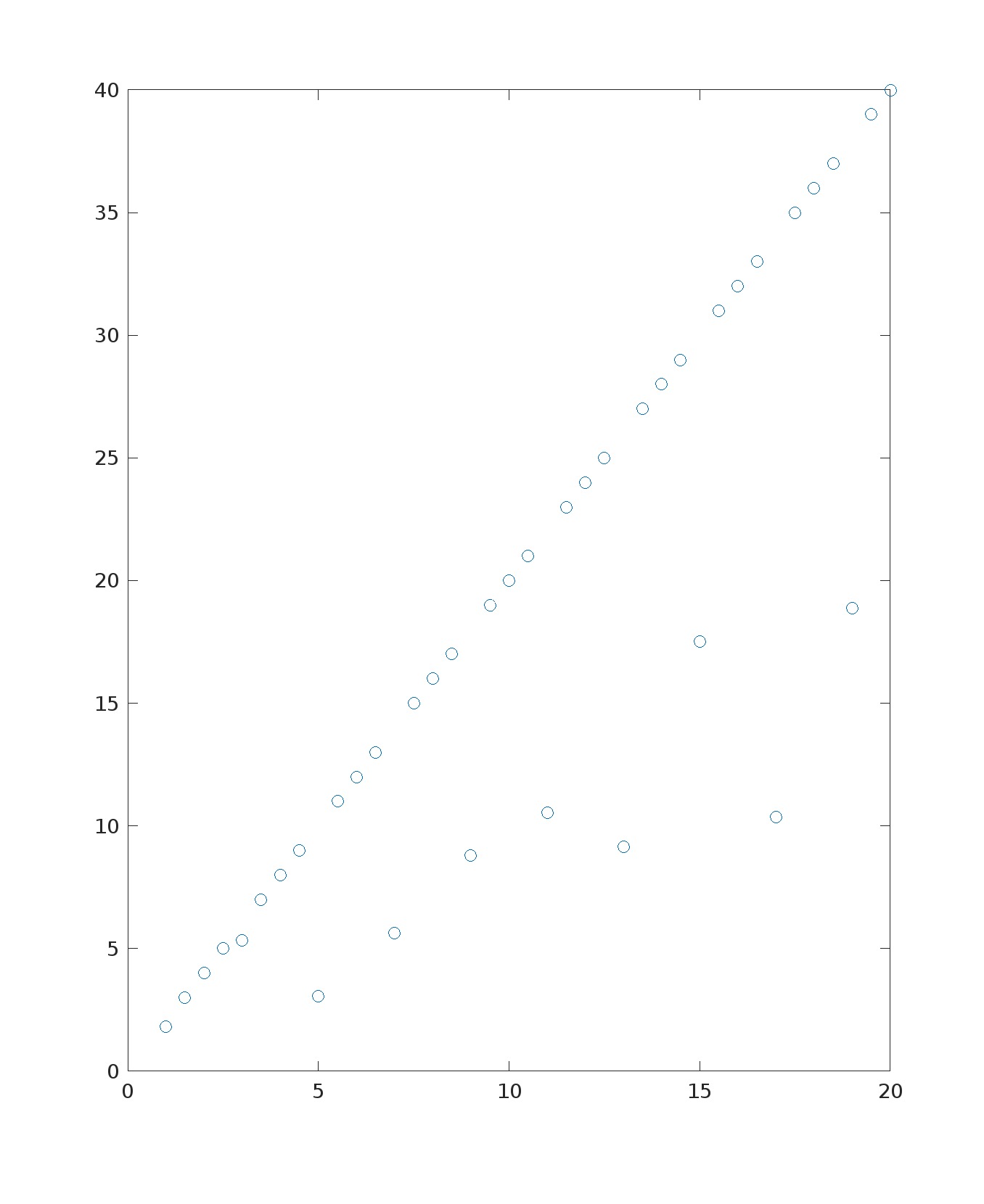
내장함수인 polyfit을 이용하여 모델을 추정합니다.
polyfit은 least square가 가장 작은 모델을 계산합니다.
아래 그림에서 확인할 수 있듯이, 이상치로 인해 원하는 모델(y = 2x)를 추정하지 못했습니다.
% fit a line using LS
fitLineFcn = @(p) polyfit(p(:,1),p(:,2),1);
p = [x', y'];
modelLS = fitLineFcn(p);
yLS = polyval(modelLS, x);
figure; hold on;
plot(x, y, 'o');
plot(x, yLS, 'r-');
legend('data', 'modelLS');
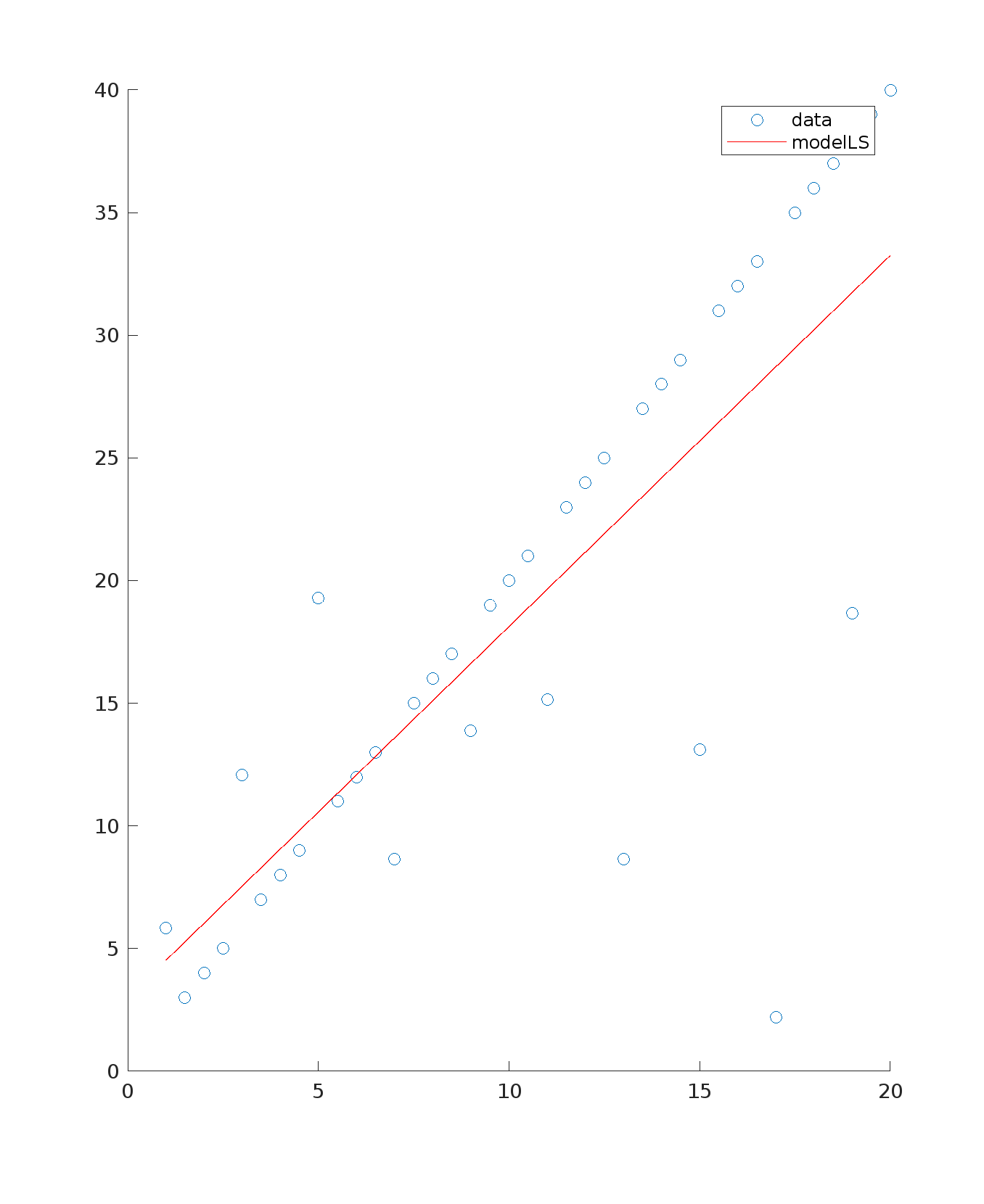
다음은 RANSAC 함수를 이용하여 모델을 추정해보겠습니다.
정확히는 MATLAB에서는 MSAC 알고리즘을 사용하고 있습니다.
간략히 차이를 설명드리면 단순히 최대 거리(max distance) 안의 데이터 수를 비교하는 RANSAC과 달리 최대 우도(likelihood)가 되는 모델을 채택합니다.
나중에 기회가 된다면 자세히 소개드리겠습니다.
코드를 보시면 랜덤하게 샘플을 뽑아(최소 sampleSize만큼)
추출된 샘플에 맞는 모델을 생성하고, 해당 모델로 모든 데이터들을 평가합니다.(evalLineFcn 이용)
이떄 최대 거리보다 작은 데이터들의 개수를 계산합니다.
해당 과정을 반복하면서 최대 거리보다 작은 데이터가 가장 적은 모델을 최적의 모델로 채택하고,
최대 거리보다 큰 데이터들을 이상치로 간주합니다.
아래 그림을 보시면 난수로 설정한 이상치들을 잘 구분하고 원하는 모델(y = 2x)도 잘 추정하는 것을 알 수 있습니다.
% Fit a line to the points using MSAC algorithm
sampleSize = 2;
maxDist = 2;
evalLineFcn = @(model,p) sum((p(:,2)- polyval(model, p(:,1))).^2,2);
[modelRANSAC, inlierIdx] = ransac(p, fitLineFcn, evalLineFcn, sampleSize, maxDist);
xIn = x(inlierIdx);
yIn = y(inlierIdx);
yRS = polyval(modelRANSAC,x);
figure; hold on;
plot(x, y, 'o');
plot(x, yLS, 'r-');
plot(xIn, yIn, 'gx');
plot(x, yRS, 'g-');
legend('data', 'modelLS', 'inlier', 'modelRS');
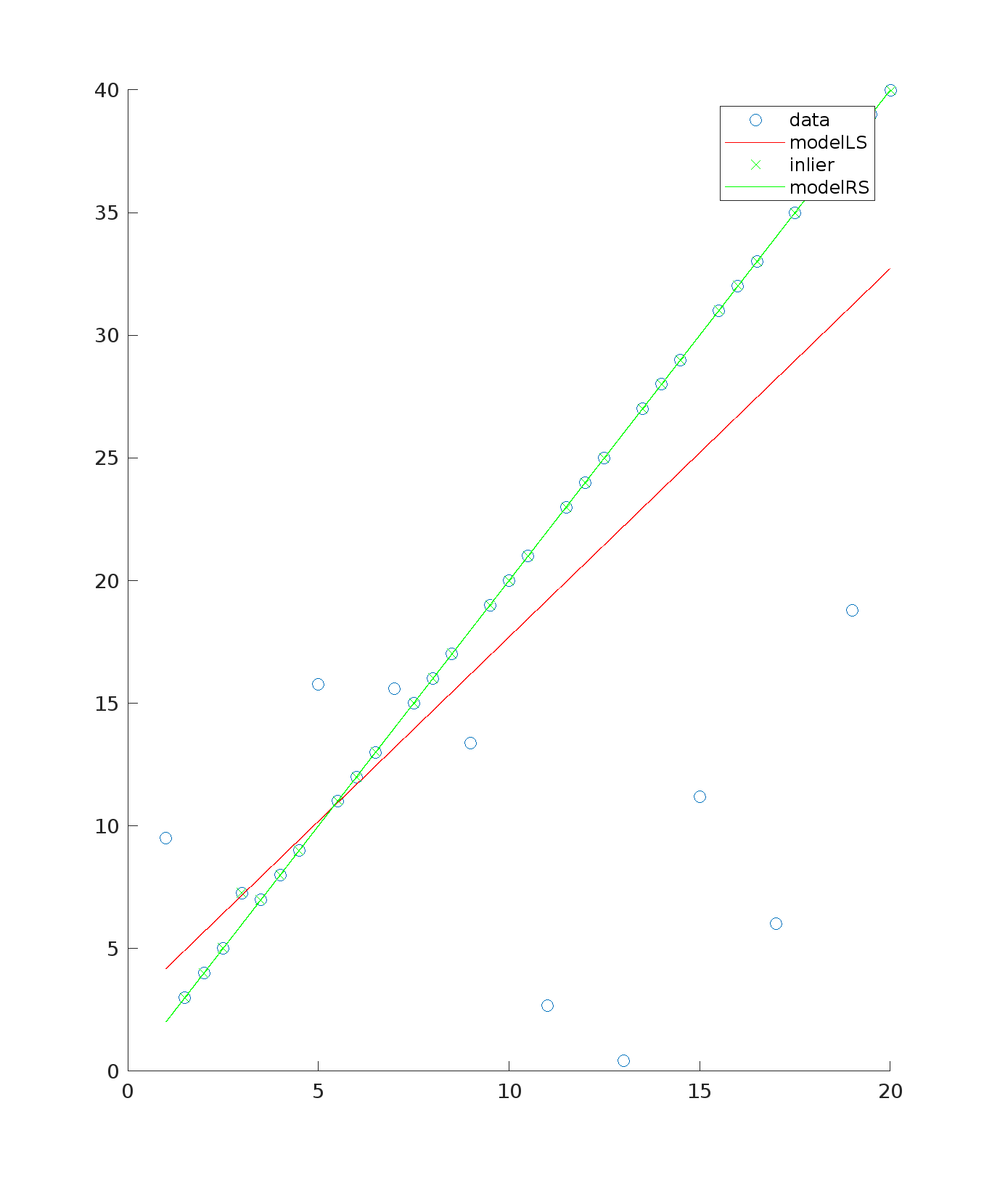
마지막으로 내장함수가 아닌 설명한 알고리즘을 기반으로 RANSAC 함수를 만들어 보았습니다.
내장 함수가 있기 떄문에 굳이 필요는 없지만 일단 해당 간단한 모델에서는 잘 동작하는 것 같습니다!
% Fit a line using my RANSAC
sampleSize = 4;
myModel = myRANSAC(p, fitLineFcn, evalLineFcn, sampleSize, maxDist)
myY = polyval(myModel,x);
figure; hold on
plot(x, y, 'o');
plot(x, yLS, 'r-');
plot(x, myY, 'b-');
legend('data', 'modelLS', 'myModelRS');
%% my Ransac
function [modelBest] = myRANSAC(p,model,evalFcn,sampleSize, maxDist)
len = size(p, 1);
maxIter = 1000;
maxCount = 0;
for i = 1:maxIter
% random sampling
sampleIdx = randi(len, 1, sampleSize);
pSampled = p(sampleIdx,:);
% estimate the model
modelEst = model(pSampled);
% evaluation
res = evalFcn(modelEst, p);
count = sum(res < maxDist);
if (count > maxCount)
modelBest = modelEst;
maxCount = count;
end
end
end
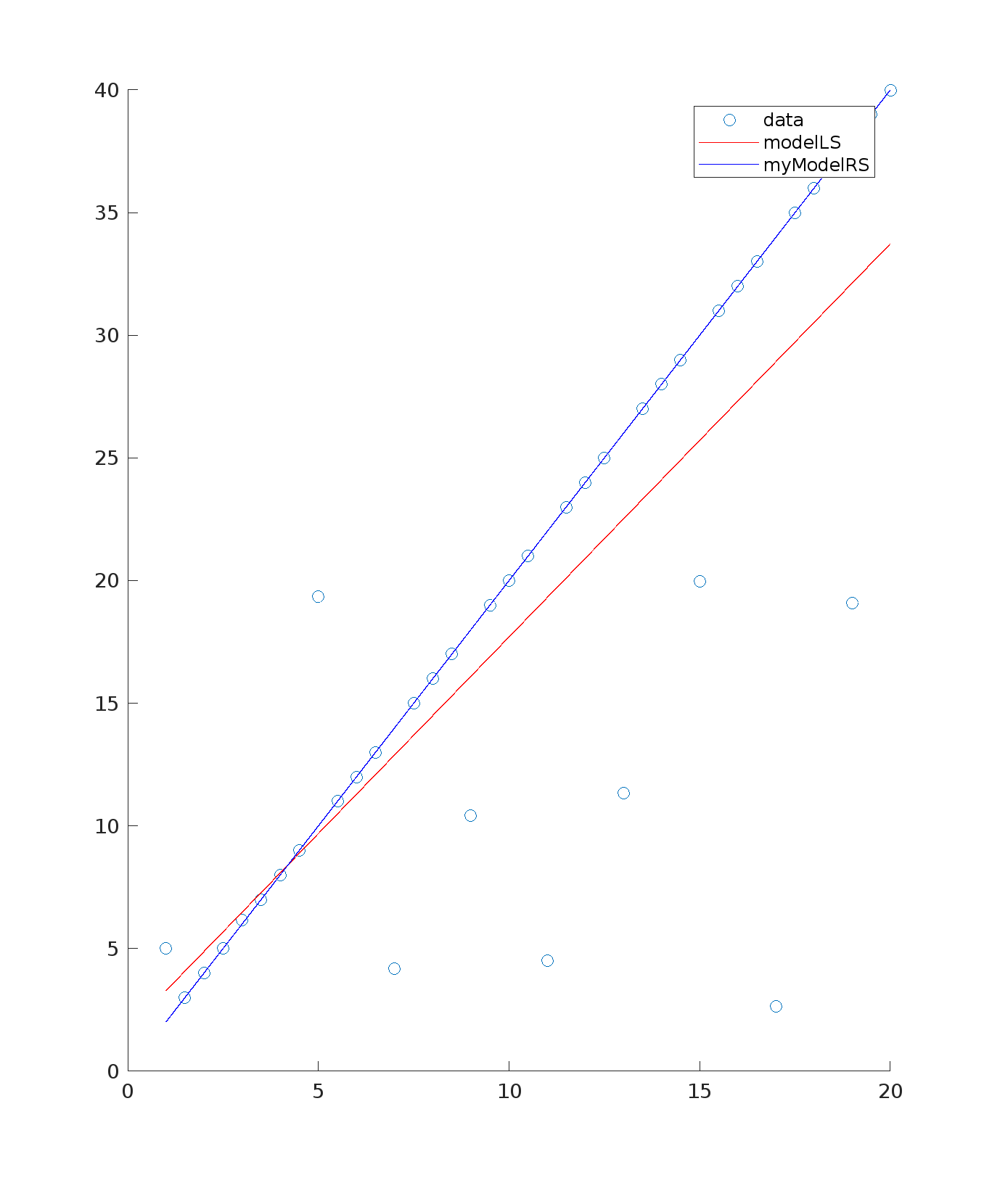
감사합니다.
'영상처리' 카테고리의 다른 글
[GStreamer] Window 10에서 GStreamer 설치 (0) | 2022.11.08 |
---|